Overview of LoFV
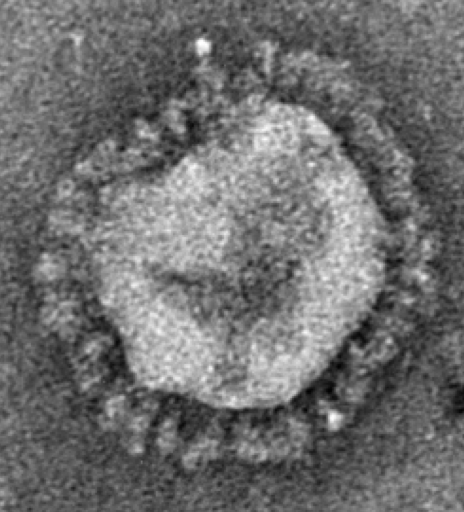
More viral sequences than laboratory data
Although deep-sequencing has uncovered thousands of animal virus sequences in nature, we still do not have an effective way to study all of these viruses in the laboratory. Basic questions linger for most of the viruses that have been discovered, including if the virus can infect humans or the animals we come into contact with. Our research approaches are aimed at annotating the ever-growing virome with functional data using a combination of synthetic biology, protein engineering and computational approaches.
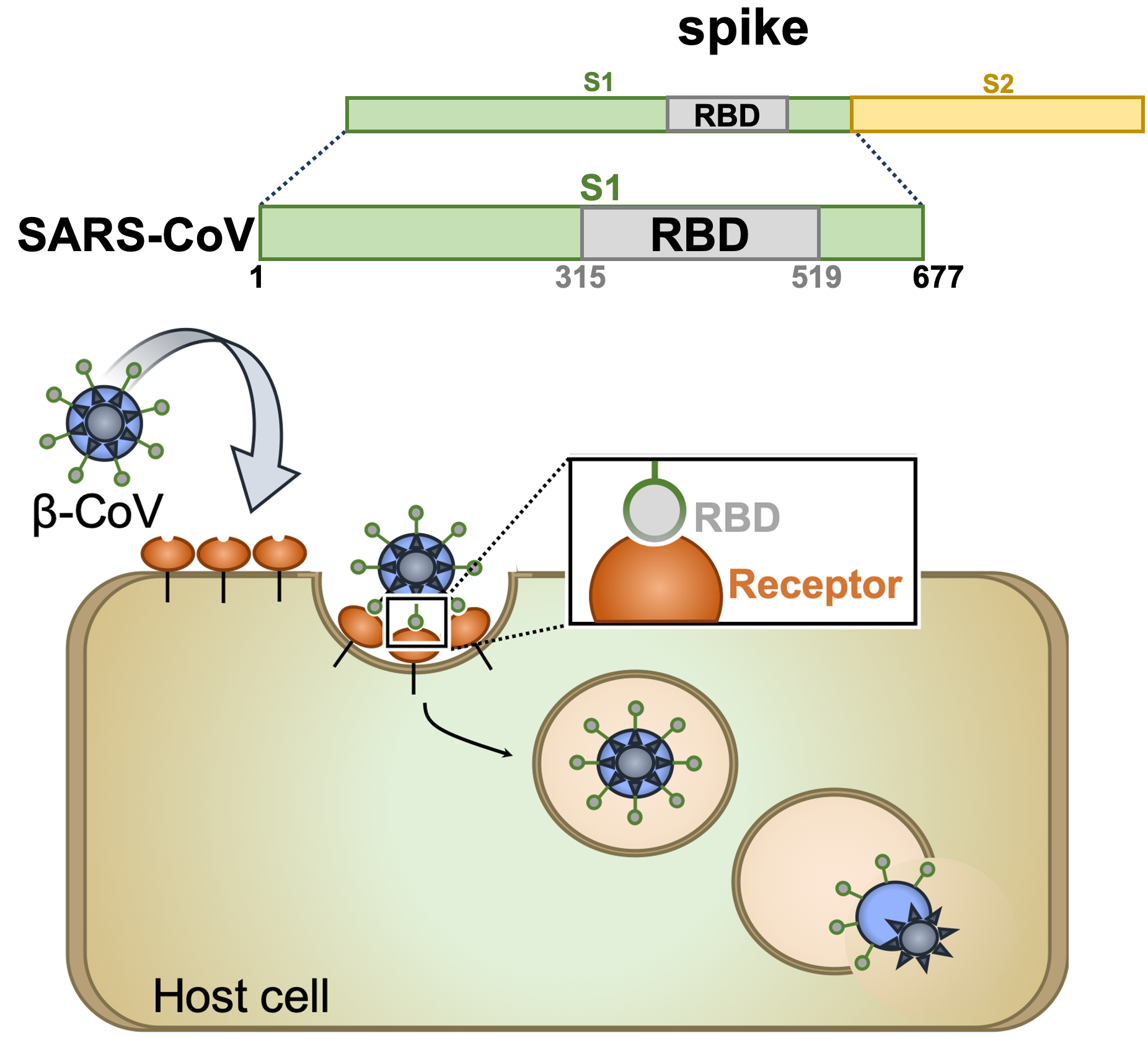
Viral entry
Viruses must first enter the cells of their hosts in order to establish an infection. Coronaviruses are covered with a viral “spike” protein that binds receptor molecules on host cells and facilitates cell entry. While the spike protein is about 1200 amino acids in length, the portion that physically contacts the cell receptor – called the RECEPTOR BINDING DOMAIN – is much smaller.
Tool building
Our work begins with developing tools that allow us to test the viral receptor binding domain (RBD) in pseudotype-viral entry assays. For the sarbecovirus subgenus, we have modified SARS-CoV spike and SARS-CoV-2 spike to facilitate rapid RBD replacement in recombinant, plasmid DNA.
SarbecoType Platform. A SARS-CoV-spike based approach to study the sarbecovirus subgenus of betacoronaviruses. Developed in 2018 for Letko et al. 2020 and used to screen sarbecoviruses for zoonotic potential.
SarbecoType II Platform. An updated, SARS-CoV-2-spike based approach to study the sarbecovirus subgenus of betacoronaviruses. Developed in 2021 for Seifert et al. 2022 and used to assess vaccinated patient serum neutralization of novel sarbecoviruses.
MerbecoType Platform. A MERS-CoV-spike based approach to study the merbecovirus subgenus of betacoronaviruses. Developed in 2021 for Letko 2024 and used to screen merbecoviruses for zoonotic potential.
SarbecoType Platform. A SARS-CoV-spike based approach to study the sarbecovirus subgenus of betacoronaviruses. Developed in 2018 for Letko et al. 2020 and used to screen sarbecoviruses for zoonotic potential.
SarbecoType II Platform. An updated, SARS-CoV-2-spike based approach to study the sarbecovirus subgenus of betacoronaviruses. Developed in 2021 for Seifert et al. 2022 and used to assess vaccinated patient serum neutralization of novel sarbecoviruses.
MerbecoType Platform. A MERS-CoV-spike based approach to study the merbecovirus subgenus of betacoronaviruses. Developed in 2021 for Letko 2024 and used to screen merbecoviruses for zoonotic potential.
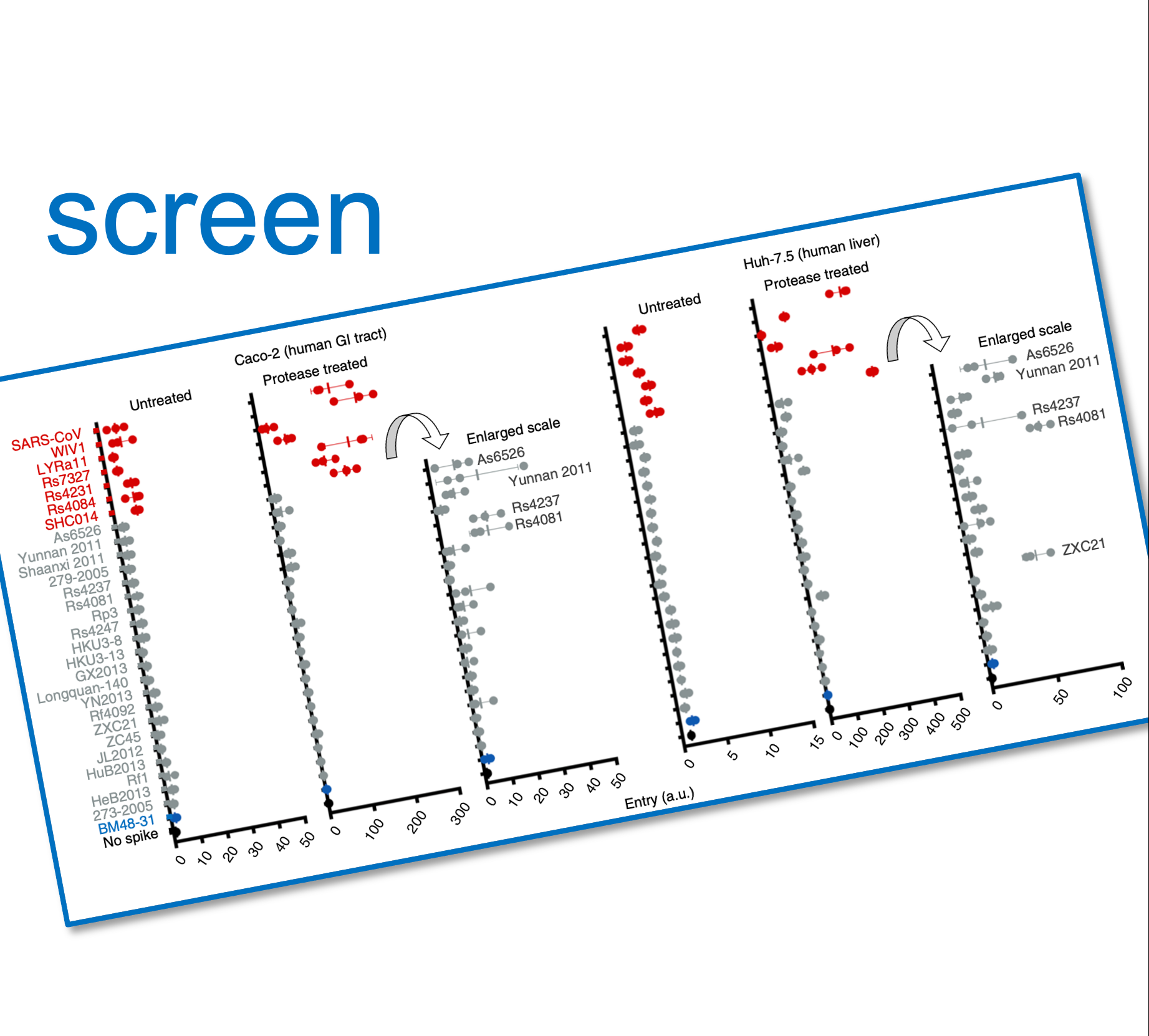
Screening coronaviruses for cell entry
We then parse sequence data from the virome to remove duplicate RBD sequences. The resulting data set is used to assemble a panel of RBDs representative of the total diversity within the given coronavirus sub-genus. Sequences from the panel are modified to allow for efficient molecular cloning into the spike-backbone plasmid. The panel is synthesized commercially as double-stranded DNA fragments and then ligated into the spike-backbone plasmid, resulting in a chimeric spike protein containing the novel RBD in place.
Our papers on this work:
Letko et al. 2020 – Functional assessment of cell entry and receptor usage for SARS-CoV-2 and other lineage B betacoronaviruses
Letko 2024 – Functional assessment of cell entry and receptor use for merbecoviruses.
For more information on this approach, check out our post with the Nature Microbiology Community
Our papers on this work:
Letko et al. 2020 – Functional assessment of cell entry and receptor usage for SARS-CoV-2 and other lineage B betacoronaviruses
Letko 2024 – Functional assessment of cell entry and receptor use for merbecoviruses.
For more information on this approach, check out our post with the Nature Microbiology Community
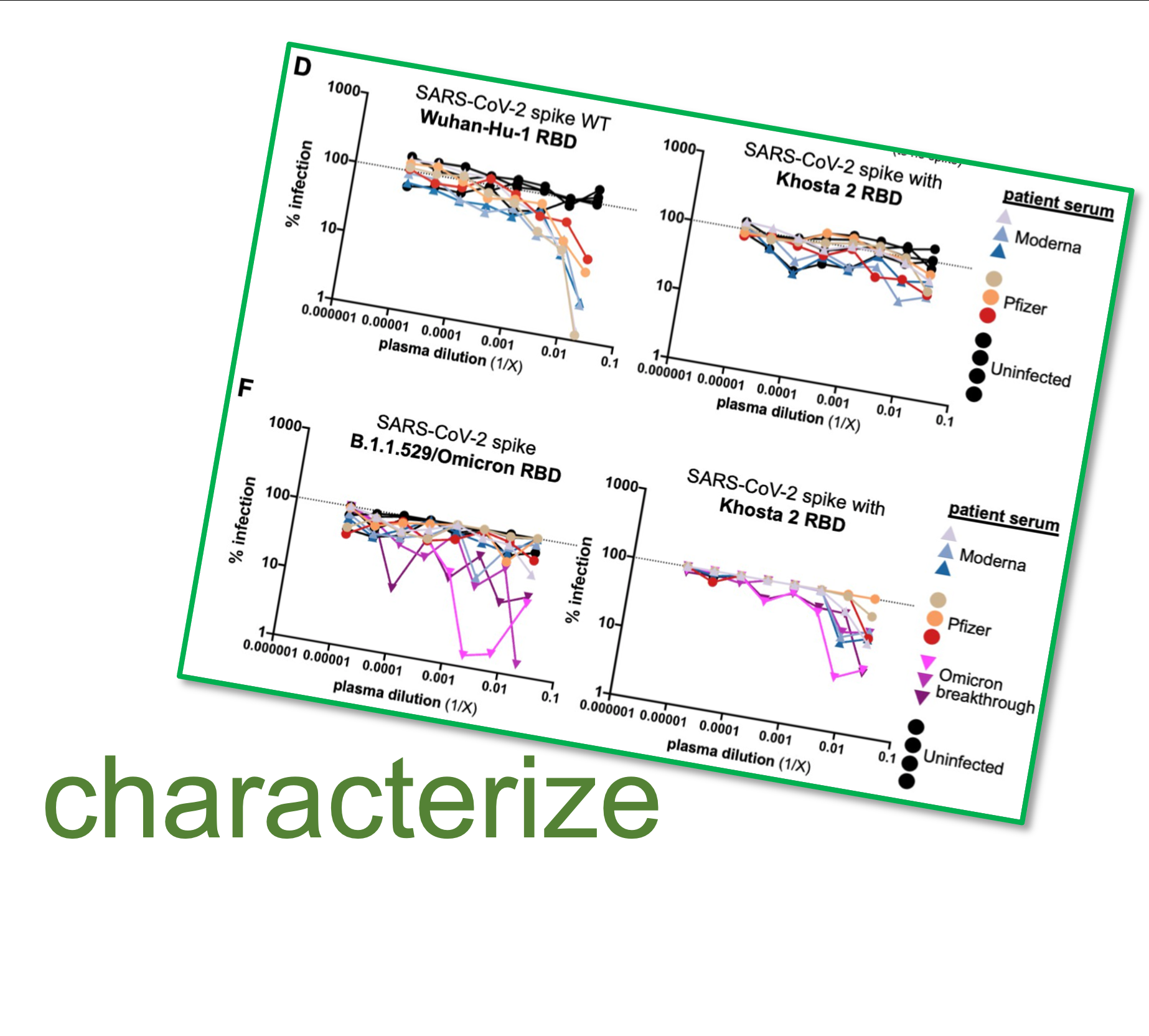
Characterizing viruses with zoonotic properties
Viruses that pose zoonotic characteristics from our screening are further studied for receptor preference and ability to evade current antiviral strategies. We also collaborate with labs developing vaccines and antivirals to help ensure future coronavirus interventions are capable of targeting these pre-emergent threats.
Our papers on this work:
Seifert et al. 2022 – An ACE2-dependent Sarbecovirus in Russian bats is resistant to SARS-CoV-2 vaccines
Seifert et al. 2022 was featured on This Week in Virology. Listen to an in-depth discussion of this work with Dr. Vincent Racaniello and the TWiV team!
Guo et al. 2022 – ACE2-Independent Bat Sarbecovirus Entry and Replication in Human and Bat Cells
Guo et al. 2023 – Isolation of ACE2-dependent and -independent sarbecoviruses from Chinese horseshoe bats
Our papers on this work:
Seifert et al. 2022 – An ACE2-dependent Sarbecovirus in Russian bats is resistant to SARS-CoV-2 vaccines
Seifert et al. 2022 was featured on This Week in Virology. Listen to an in-depth discussion of this work with Dr. Vincent Racaniello and the TWiV team!
Guo et al. 2022 – ACE2-Independent Bat Sarbecovirus Entry and Replication in Human and Bat Cells
Guo et al. 2023 – Isolation of ACE2-dependent and -independent sarbecoviruses from Chinese horseshoe bats
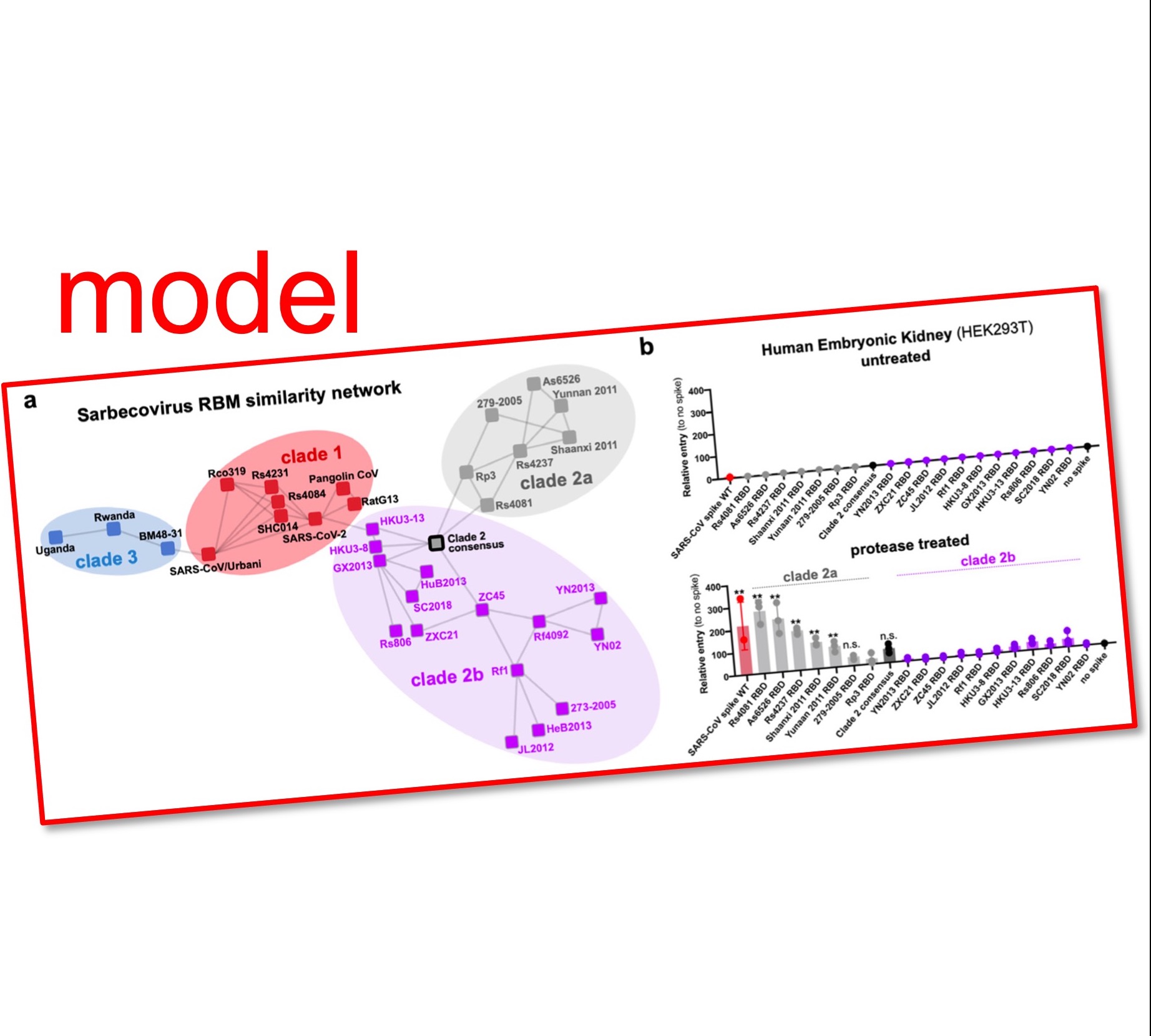
Model the data to find trends
More viruses will continue to be discovered. More than 70 new animal-derived sarbecovirus genomes were published in the last year. Thus, testing every new virus sequence – while possible with our approaches – is not practical. We are collaborating with computational biologists to model larger trends from our screening and characterization data. With enough laboratory data fueling advanced machine-learning models, we will be able to predict viral zoonotic risk from sequence without the need for laboratory tests.
Our papers on this work:
Khaledian et al. 2022
Our papers on this work:
Khaledian et al. 2022